To keep pace with other sectors that have already adopted digital practices and artificial intelligence (AI), the fashion industry is facing an unprecedented challenge to digitize its processes—starting with textiles. In this guide, get to know:
- How artificial intelligence can help the fashion and apparel industry create digital textiles.
- Why digital apparel design workflows must start with accurate digital simulation of textiles.
- How SEDDI’s AI is validated to ensure high-quality digital textile outputs.
The Challenge of Digitizing Textiles
Digital textiles are the building blocks for digital apparel design workflows, for marketing and selling textiles online, and for online marketplaces and material platforms. Fabrics are particularly difficult to digitize, being complex materials with multi-scale components affecting both appearance and drape, and the fast pace of the actual industry requires the new digital workflows to be scalable, simple, and traceable.
While existing lab devices can obtain different properties of textiles—optical gonioreflectometers, or machines for mechanical testing—no existing solution can handle all of them jointly. Current workflows require several manual steps, creating roadblocks for scalability, repeatability, speed, and consistency.
This is no longer the case. SEDDI has developed a solution for holistic digitization of textiles that creates realistic digital copies in minutes from a single image, with minimal computing cost, scaling up to the needs of brands and retailers. This technology is a key component of our SEDDI Textura software.
How is Artificial Intelligence Used in the Fashion Industry?
SEDDI’s textile digitization is grounded in modern machine-learning algorithms. Our team of scientists have developed a large database of textile materials digitized—down to the yarn and fiber level—with proprietary precision equipment for optics and mechanics. Using this data, we have trained models that predict these properties, requiring just a single image of a desktop scanner as input, and some metadata invaluable for disambiguating some cases. Thanks to our highly precise database of textiles, our solution can infer properties that expand beyond the visible features in the scanned image. From a research perspective, SEDDI is the first to develop a method to leverage a single image to produce such type of data.
How is the Technology Validated?
At SEDDI, we validate our digitalization processes using strict scientific principles and methodologies. Our scientists conduct quantitative and qualitative validations using two methods: per-parameter validation and in-context validation.
Per-parameter Validation
The digitalization parameters obtained using our proprietary optical and mechanical testing hardware serve as ground truth data. To train our artificial intelligence we split this data into two distinct sets, training and testing, and measure the error of our final model on the test set to validate the generalization capabilities of our method. We have a number of error metrics to quantify the estimated error, which measure the difference between ground truth and estimated parameters.
In-context Validation
Per-parameter validations (see above) are useful indicators to assess the model’s performance, compare models, and track its evolution over time. However, sometimes they are not enough to provide a perceptual understanding of the error: different sets of parameters might convey the same digitalization result (visual appearance or drape). Our in-context validations aim to overcome this issue.
In this process, our team simulates and renders specific scenes using the full set of digitalization parameters that showcase certain aspects of the fabric materials, which are different for optical and mechanical validation. Further, we generate the same scenes with simulation engines and compare the output with our internal methods and real scenes. Some of these comparisons are currently done qualitatively, although we are developing methods to provide numerical ratings that correlate with human perception.
Optical Validation
- Per-parameter Validation
We evaluate the accuracy of the optical estimation (i.e., texture maps) using several metrics commonly used in scientific methodologies.
In particular, we evaluate the per-pixel/per-map performance using two metrics. The first metric is the Mean Absolute Error (MAE) and its Standard Deviation, which measures absolute precision per map. In this metric lower values are preferred. The MAE for the normals measured in degrees is 2.1 ± 1.7. The MAE for specular, roughness, displacement, and alpha (measured in a range between 0 and 255) is 23.2 ± 23, 14 ± 12.8, 12 ± 10.2, and 5.1 ± 7.7, respectively. The second metric is the Spearman correlation coefficient, which measures the relative error; this accounts for situations where there is more than a single solution. In this case, values greater than 0.7 mean a significant correlation (which is preferred), and a value of 1 is a perfect correlation. We obtain 0.8, 0.7, and 0.9, for specular roughness and alpha.
- In-context Validation
In another set of experiments, we evaluate the quality of the maps directly in a rendered scene. We compare ground truth (GT) renders obtained from our gonioreflectometer (SEDDI’s proprietary optical capture machine) with AI renders estimated from SEDDI Textura.
- High Quality:
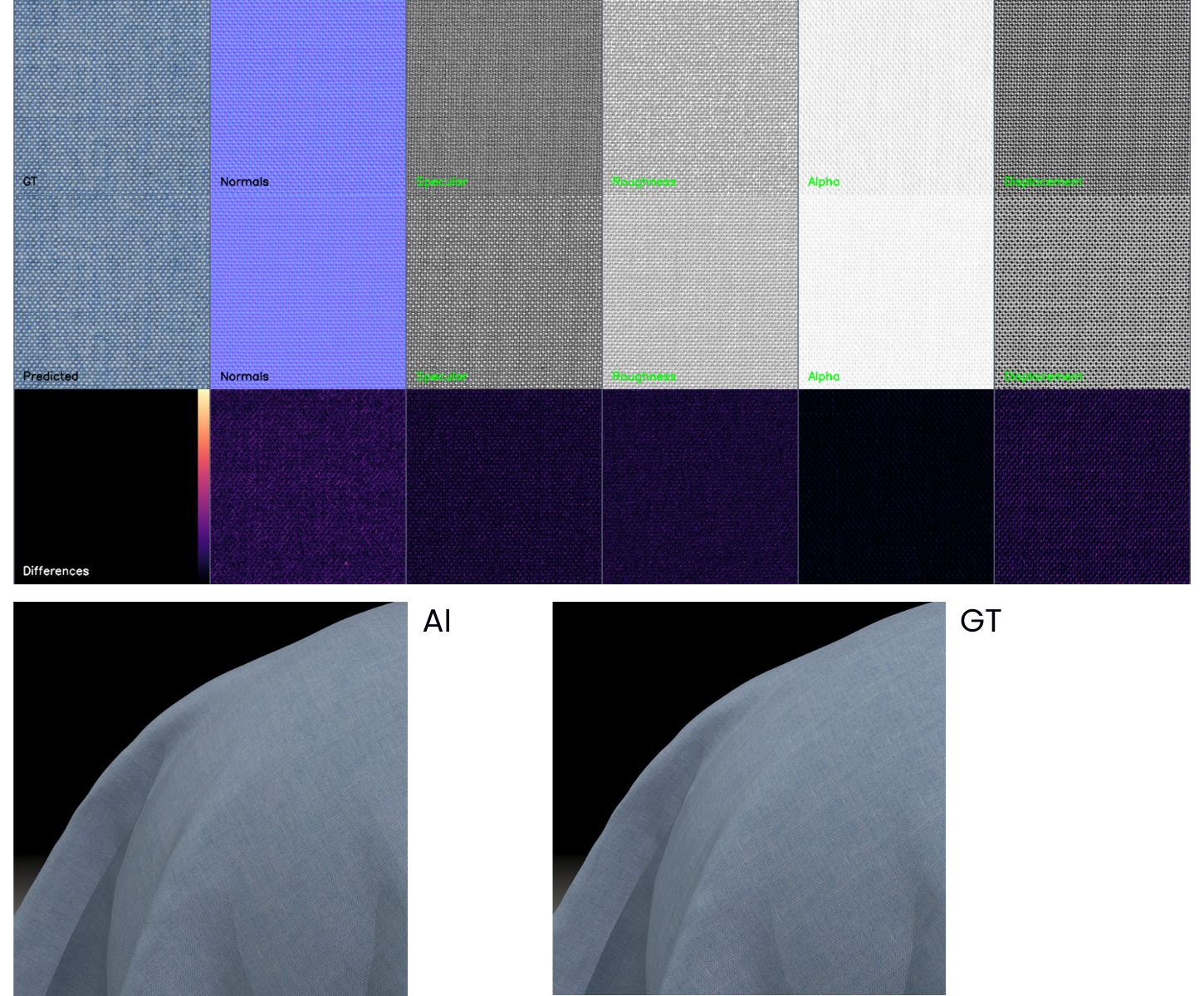
1. Comparative ground truth (GT) renders obtained from our gonioreflectometer with AI renders estimated from SEDDI Textura.
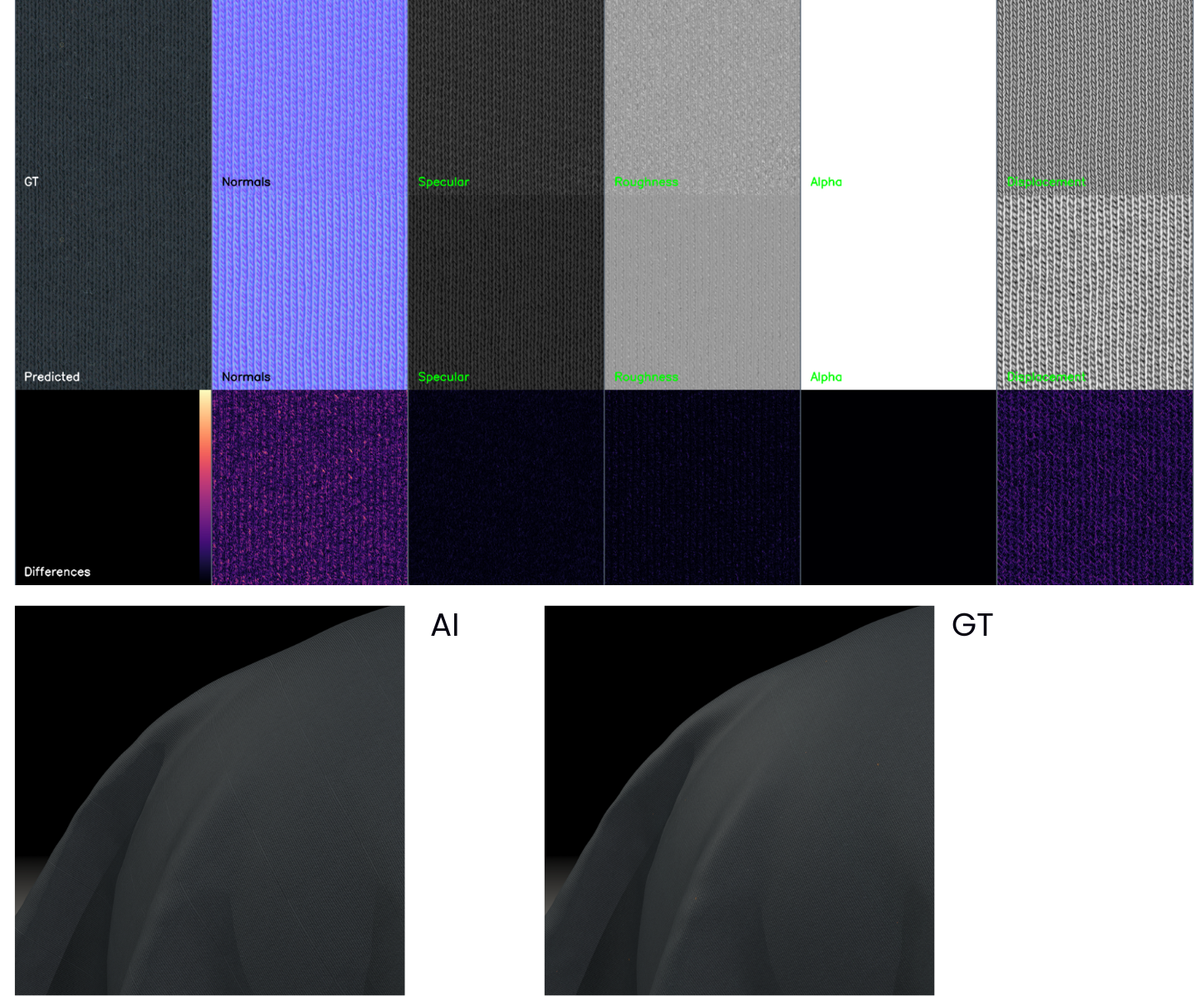
2. Comparative ground truth (GT) renders obtained from our gonioreflectometer with AI renders estimated from SEDDI Textura.
- Lower Quality:
Our AI showcases lowest performance on satin fabrics, fabrics with mesostructure, and holes.
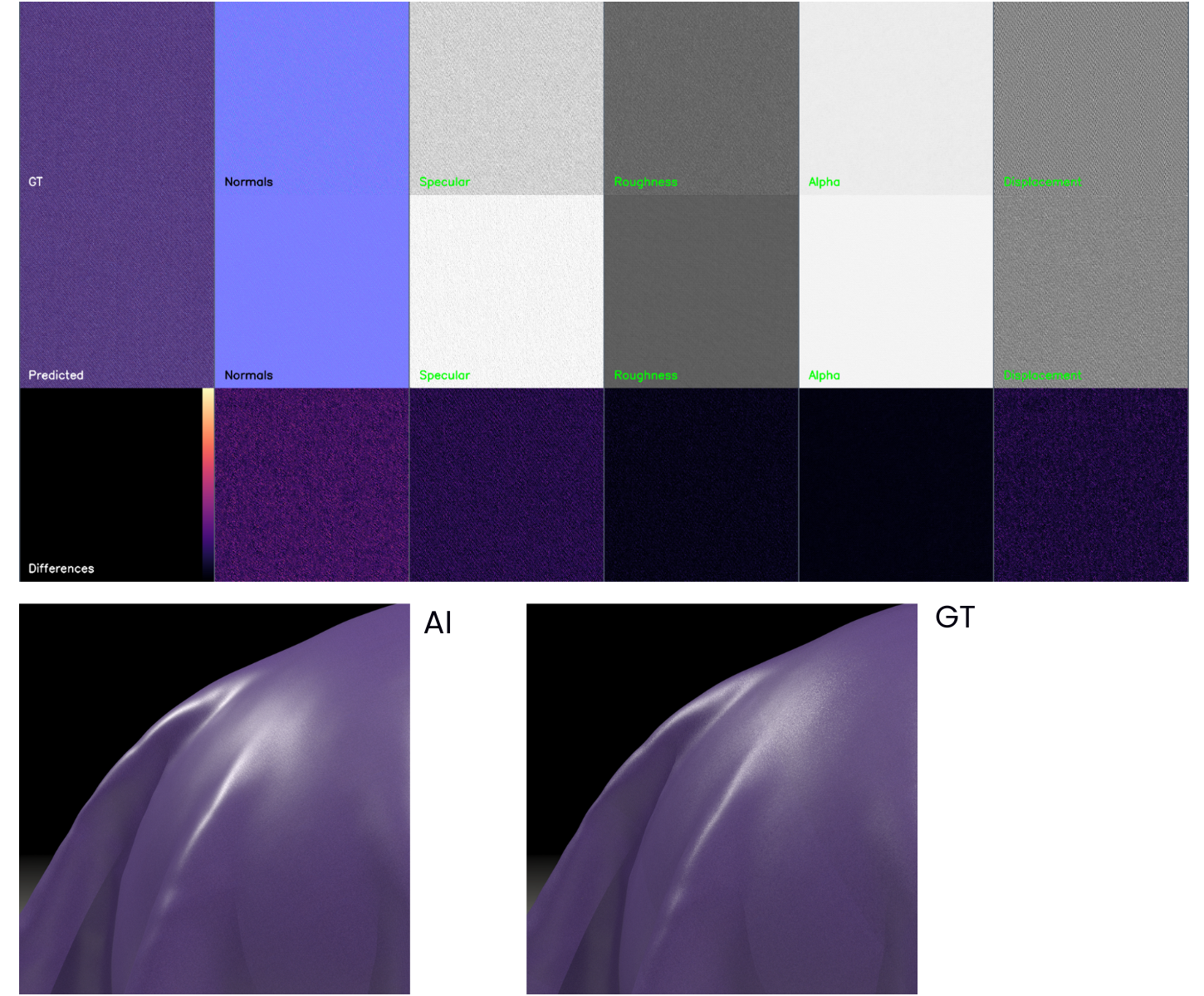
3. Comparative ground truth (GT) renders obtained from our gonioreflectometer with AI renders estimated from SEDDI Textura.
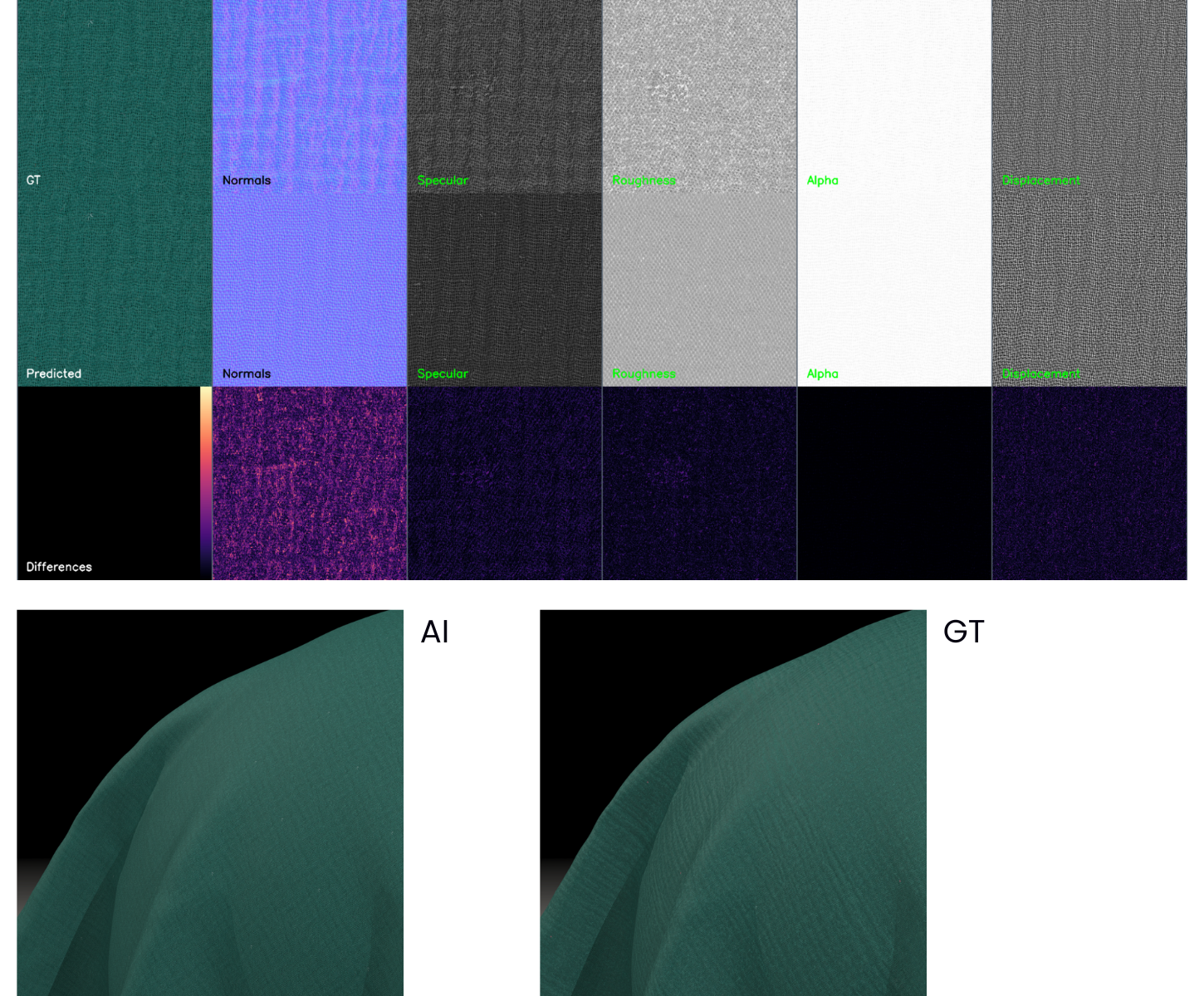
4. Comparative ground truth (GT) renders obtained from our gonioreflectometer with AI renders estimated from SEDDI Textura.
Mechanical Validation
Per-parameter Validation
Textura generates mechanical parameters for different third-party 3D fashion design solutions that leverage digital textiles in their software, such as Browzwear and CLO. We evaluate our numerical accuracy on each parameter of each third-party solution using several standard metrics. A particularly informative and intuitive metric is the normalized mean absolute error (NMAE) and its standard deviation (STD). The NMAE measures the absolute difference between ground truth and estimated parameters, and divides it by the effective range of the parameter to enable comparison between deformation modes—where parameters can have very different scales—and across multiple software. We test our model on hundreds of fabrics. Using this metric, and for 90% of the fabrics we tested, the NMAE for Browzwear parameters is 9% for both stretch and bending, with a STD of 9%. For CLO parameters, the error is 11% for bending and 12% for stretch, with a STD of 13% for both.
These errors are quite reasonable and the visual difference in drape is usually insignificant when compared to ground truth. Textura’s mechanical service has also been evaluated by third-party textile engineering experts, which have reached similar conclusions and have validated the accuracy of the drapes against ground truth data using the standard Cusick Drape Test.
In Context Validation
In addition to per-parameter validation, we leverage in-context validation to evaluate the actual perceptual impact of our estimation error. In the case shown below, the scene used is a drape of a fabric on a sphere. Our fabric sample is square, measuring 50x50cm, and it is draped on a sphere with a diameter of 25cm. This setup allows the fabric to express the full range of parameters. We evaluate our perceived error in this setup by surveying a qualified group of people familiar with 3D simulation software, and ask them to evaluate the similarity of the ground truth parameters to the output of the AI estimation on a Likert scale.
- High Quality:
- Lower Quality:
The Future of AI in the Fashion Industry
This practical and necessary application of AI is a great example of the future of AI in the fashion industry. It is impossible to digitalize fashion and apparel design and manufacturing workflows, without first digitalizing the foundation of garments; the fabric.
SEDDI’s Textura solution delivers much greater speed, high quality, and fingertip convenience at a reasonable cost. No more 4-week lead times for lab results, no high-priced service for texture maps and physical properties, no $30-40k investment in hardware, and no investment in skilled 3D lab technicians.
Want to experience Textura’s AI for yourself? Try it for free today.